Using AI for surgical planning
In today's OR management, resources are limited and many variables complicate scheduling. Using artificial intelligence (AI) technology, Klinikum Stuttgart significantly improved OR utilization. The stability of the schedule increased employee satisfaction, as regular working hours were more adhered to. [1]
Effects of AI in OR planning at Klinikum Stuttgart
-
39% increase in correctly planned surgeries
-
30% higher accuracy compared to standard duration
-
6% improved room utilization within the core operating time
The challenges of OR planning
Accurate estimation of operating time is one of the most important factors for optimizing OR utilization. Research has shown that subjective estimates of surgical procedures can be off by as much as 50%. [2]
This is why most hospitals with surgical units experience incorrectly estimated surgery times. This leads to short notice changes due to medical emergencies or reduced staff resources.
The average mean deviation of the planned incision-to-suture time from the actual time prior to the introduction of AI, was 19 minutes – due to the fact that Klinikum Stuttgart already had standard values for surgery time statistically derived from historical data.
It is our goal to organize surgical interventions in such a way that we pave the way for optimal resource and capacity utilization, well-regulated working hours for our staff and adherence to schedules for our patients.
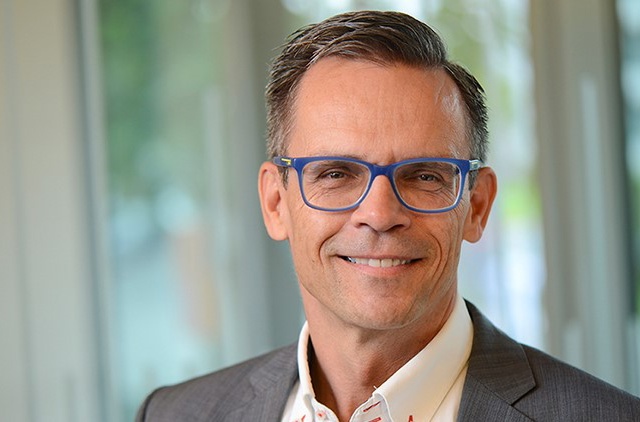
Better OR management through machine learning
In 2012 it was already shown that estimating the operating time based on documented data and context data, can increase accuracy by up to 36%. [3] Aiming to increase the planning accuracy of surgical procedures, Klinikum Stuttgart started using artificial intelligence in Torin OR Management solution in November 2021.
The AI algorithm [4] [5] takes into account pre-selected parameters that influence surgery times, based on objective data. AI analyzes estimated and actual times of previous surgeries and puts them in context with 27 different variables. It then spots dependencies between variables and generates patient-specific predictions for each intervention type with increasing accuracy over time as more data becomes available.
How much time should be accurately calculated for surgery on a patient over 65 years of age or how much time should be added or subtracted for each surgeon based on his or her individual skills (prediction scenarios based on the actual duration of surgery as opposed to algorithm-based planning are exemplified in the figure above).
30% more accurate than standard surgery duration
A total of 4,225 surgeries were scheduled using AI between November 2021 and May 2022. Utilization was balanced among departments, but gynecology, general surgery, ophthalmology, and urology used AI most frequently.
On average, the AI prediction is 30% more accurate than the average estimated surgery duration stored in the master data. The master data was determined from the 75% percentile of the actual surgery times of analog procedures performed to date.
In contrast to manual planning (which is usually done in time units of 0.5-1 h), AI plans to the minute, and with remarkable accuracy. The very first time Klinikum tested it, the AI was off by a single minute – compared to the surgeon's estimation, which was off by 22 minutes.
Graph over estimated duration versus actual duration. The estimated duration compared to the actual duration is closer to the ideal line with AI. A: AI duration. B: Standard duration.
39% increase in correctly planned surgery
The use of AI allowed for more accurate OR scheduling (by18.4%), yielding a 39% increase in correctly scheduled surgeries.
Surgeries with excess hours took 7.3% less time on average and the planning accuracy with regards to undertime was improved by a substantial 30.3%. On average, 6.8 minutes could be gained per surgery, adding up to a significant overall increase in OR utilization and employee satisfaction.
We were particularly surprised by our very first surgery using AI prediction, a carotid TEA in vascular surgery. This was planned by the surgeon to take 90 min, by the AI to take 111 min, and was ultimately performed in 112 minutes.
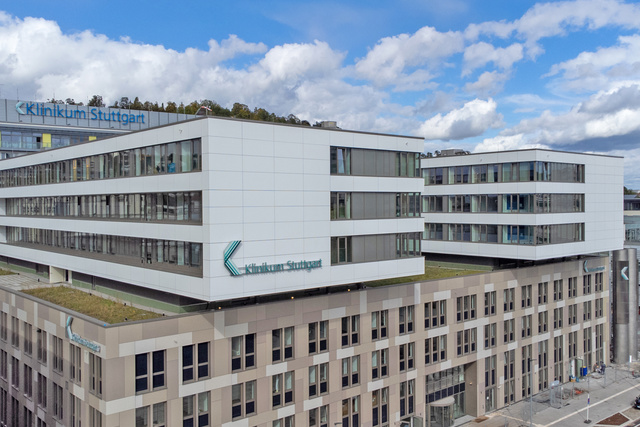
OR utilization improved by 6%
Using AI based on documented and contextual data enables better estimation of actual surgery times. The time savings accumulate, yielding an improved OR utilization and better kept schedules.
The 30% higher accuracy of the AI compared to the standard duration is particularly remarkable because Klinikum Stuttgart had very good historical master data to begin with, a fair assumption to make would be that hospitals with less stringent planning will see even greater improvements.
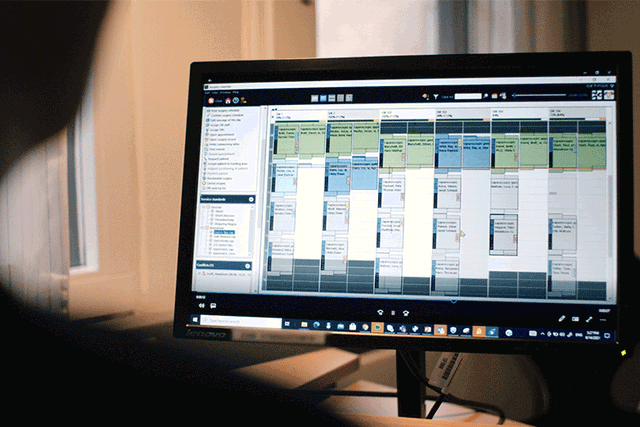
AI machine learning at Klinikum Stuttgart
In developing the machine learning model, Klinikum Stuttgart relied on surgical data from several years (>50,000 surgical procedures). Only surgeries performed 100+ times were included in order to guarantee a sufficient amount of data. Whenever input data changed, surgery times updated individually in real time – including scheduled surgeries yet to be performed. The actual calculations took mere fractions of a second, even with hundreds of variables, as the machine learning model was applied rather than retrained.
As a result of the introduction of AI, Klinikum Stuttgart obtained more precise surgery time estimates and anesthesia setup times individualized for each patient, while all influencing variables were transparently displayed.